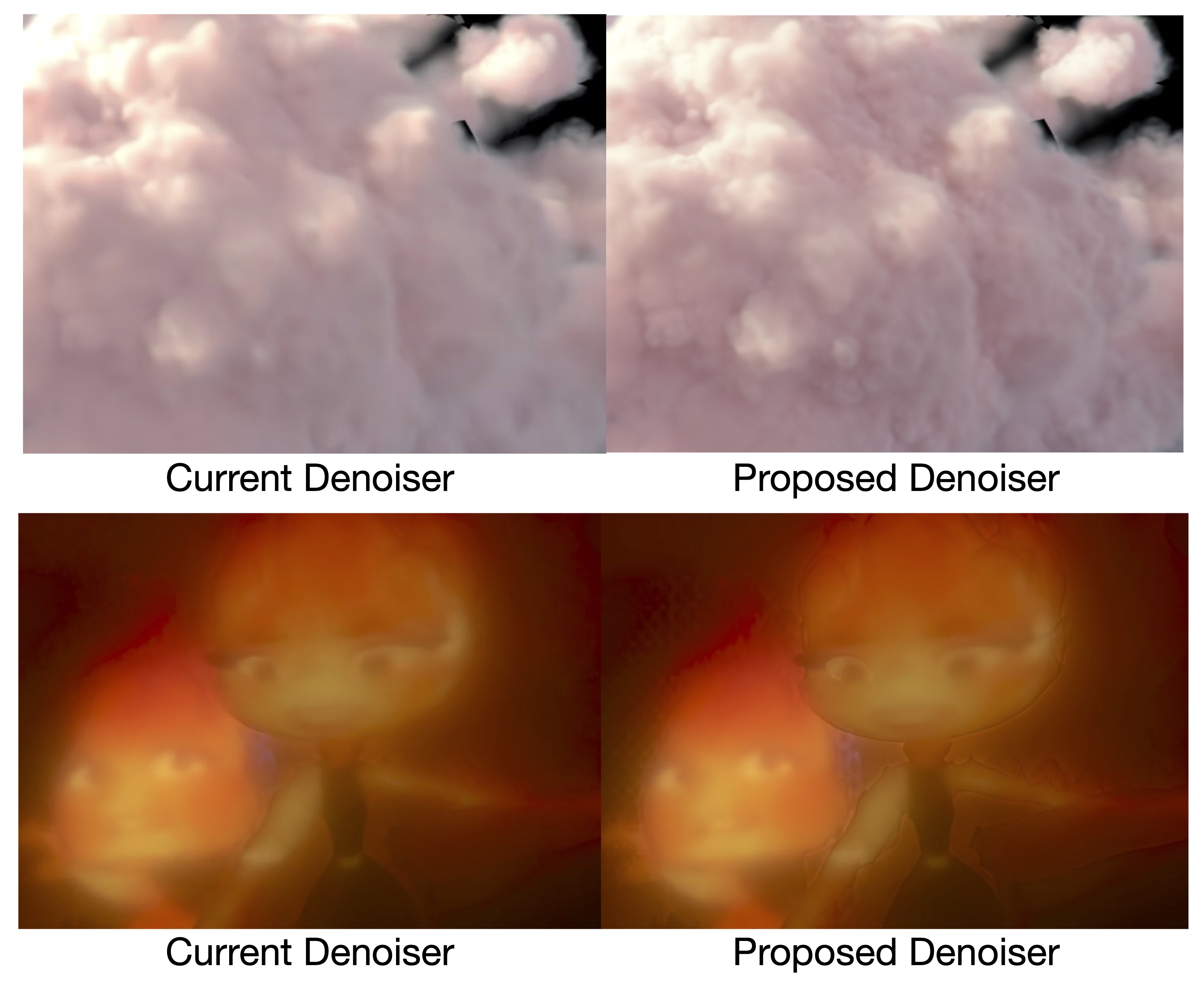
Shilin Zhu, Xianyao Zhang, Gerhard Rothlin, Marios Papas, Mark Meyer
Abstract:
Denoising is an integral part of production rendering pipelines that use Monte-Carlo (MC) path tracing. Machine learning based denoisers have been proven to effectively remove the residual noise and produce a clean image. However, denoising volumetric rendering remains a problem due to the lack of useful features and large-scale volume datasets. As a result, we have seen issues such as over-blurring and temporal flickering in the denoised sequence. In this work, we modify the production renderer to generate many types of potential volume-specific features that might improve the denoising quality, and then run a state-of-the-art feature selection algorithm to detect the best combination of those features. To train the denoiser for production use, we collect thousands of unique volumetric scenes from our recent films, and augment the inputs to create a large dataset for training. Our evaluation shows a good amount of quality gain compared to the version currently in use.
Paper (PDF)
SIGGRAPH Talks 2023